We’ve all heard the buzzwords Big data, Data Analytics, Metadata, and Teradata. But what do they all mean? Most enterprises today have avenues to collect tons of data. However, the data does not become meaningful unless analyzed and organized. This is where Data Analytics comes in.
Simply put, it is the process of examining raw data to draw out meaningful, actionable insights, which support informed data-based decisions. Most enterprise businesses have departments or teams that help with this – typically, it’s a group of researchers, data scientists and analysts that use varied tools to crunch data and gather insights.
Data Analytics has quickly become a critical piece to any successful enterprise because it helps reveal valuable information about the organization and its consumers that could otherwise be unknown or ignored.
Influence in product management
It is only natural then, that product managers should harness this power to make better decisions about their products. Data analytics is one of the most important yet frequently overlooked aspects of successful product management. To be sure that your new product will be well received, it's vital to understand how you can use data analytics to study and analyze the market in which your product will be sold. Although often overlooked, it’s critical to every step of the process from conception to launch and beyond.
What data should a Project Manager look at?
These days, there is an ocean of data that you can collect about your product. The real challenge is in identifying the right data streams to inform your product strategy. In most cases, product managers tend to look at three different datasets – user research data, product analytics, and market research data.
User Research
User Research is paramount to the development and maintenance of any product. This includes user surveys, A/B testing, user interviews, and pretty much anything else that can help you gather data from your users. The intent is to help you understand how users use your product, what their behaviors are, what they are enamored by, and what is likely to keep them engaged. Equally important to note is that this also helps get rid of any preconceptions you may have about the product or the user. Getting rid of those assumptions is crucial to the success of any product.
Product Analytics
This is when tracking tools like Google Analytics prove invaluable. I have worked with several clients who thought they had their product figured out to a tee but were shocked to see that several of their deductions and assumptions were contradictory to how consumers actually used their product. Leveraging a tool like GA reveals a wealth of insights, unveiling undiscovered vital information about how your users interact with your application. Measurements like user abandonment rates, conversion channels, bounce rates, and engagement rates, are all valuable data points that could adversely change your product roadmap and affect feature prioritization.
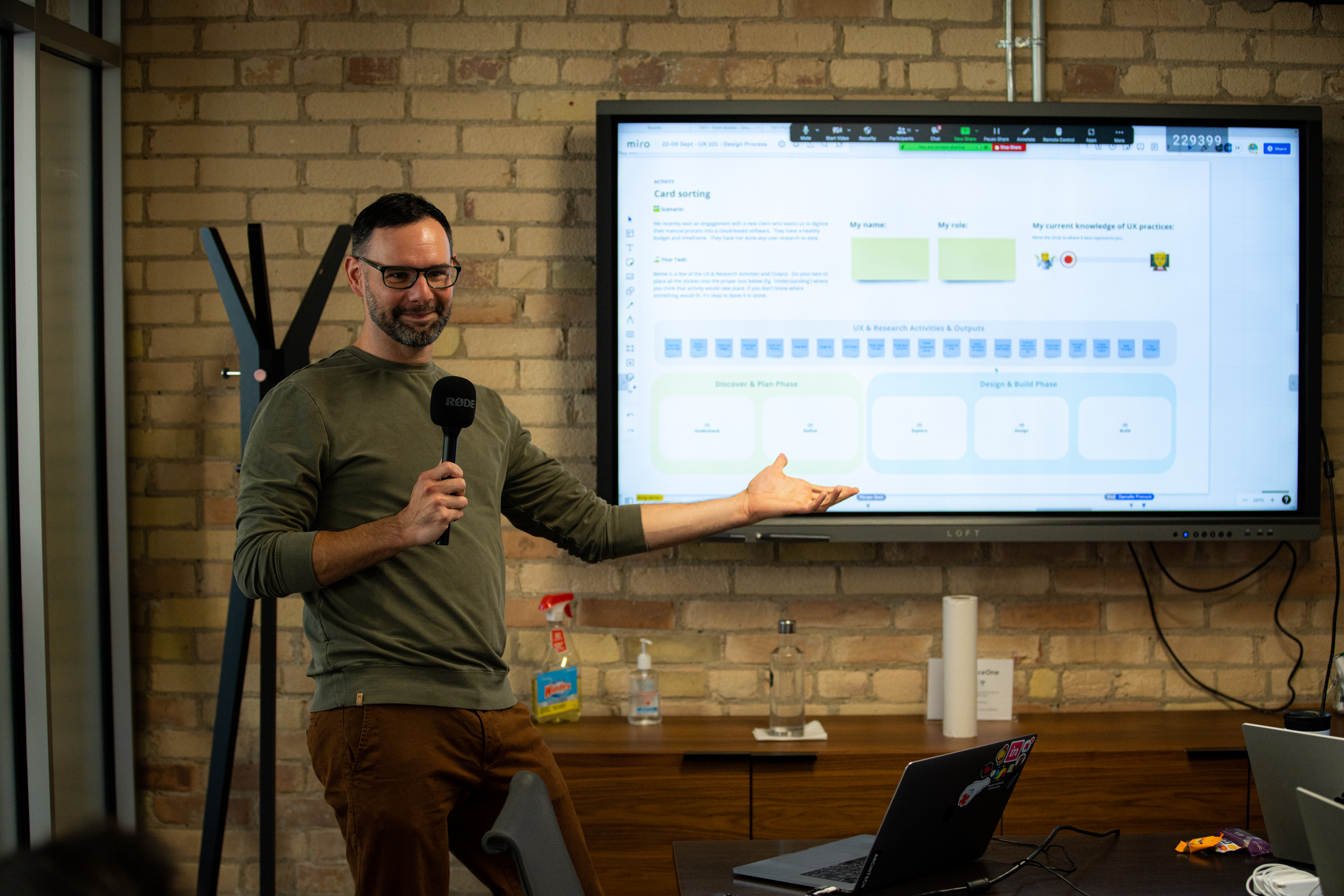
The primary product analytics for an organization vary depending on the nature of its business. This could be user conversions, user retention rates, traffic sources, or demographics. You could even set up acquisition funnels to understand where users are exiting your application and concentrate marketing efforts on the right audience. Examining the right product analytics can help divulge new avenues for innovation that could set your product apart from the rest of the competition. Simply put, product analytics helps to make better product decisions.
Market Research
There is a common misconception that the market is a projection of your user base and that market research yields the same observations as user research. This is only partly true. There are similarities between the two, in that they use similar techniques to gather information (surveys, focus groups, interviews, etc.) to expose and/or verify assumptions and test hypotheses.
However, the key difference is in the outcome of the two activities. User testing revolves around how users use your product, which supports user experience and product design. Market research is aimed at studying your competition and understanding the environment in which your product needs to thrive.
Observing your user base alone cannot generate that information. This needs competitor analysis, target market identification, studying market trends, analyzing customer buying habits, and working on activities that help you understand how your competitors are performing. In uncovering these data points, you find the best way to differentiate your product in the market. Market Analysis is as crucial to setting your product up for success as user testing or gathering product analytics.
Qualitative vs. Quantitative Data
There is also the ever-present debate about what matters more - qualitative or quantitative data. Quantitative Data is more popular because hard numbers are easier to understand and assimilate than soft data like user reviews.
I confess I prefer qualitative data. I like to look at cold, hard numbers - NPS scores, app bounce rates, user conversion percentages, and so on - rather than rely on open-ended metrics like user observations, focus group decisions, or behavioral findings. Some research has shown that most organizations prefer quantitative data to qualitative data because it is objective and easily understandable.

In truth, product managers need both types of data to paint a full picture and ensure that the data gathered is both complete and accurate. During the initial stages of product development, qualitative research can give fuzzy product concepts more clarity.
Exploratory product research, feature refinement, and post-launch reviews are all key qualitative research tactics that help discover unanticipated insights that will pave the way for ideation and future research. This needs to be coupled with key quantitative metrics to help understand user behaviors and make more effective decisions.
Challenges
With all the possible benefits that employing data analytics offers, product managers need to be wary of the challenges this brings. Product managers today have a wealth of data available to them, and it becomes tricky to decide which ones to pay attention to and which ones to deprioritize.
Using data analysis tools such as PowerBI and Tableau can massively help to consolidate and organize this data better. However, you still need to utilize your product understanding to decide which datasets are useful and which ones can be ignored. With the proliferation of artificial intelligence and AI-focused tools around us, platforms like Akkio, Polymer, and MonkeyLearn are all rapidly gaining traction in helping users visualize and rearrange data to suit their needs and interests.
While prioritizing datasets is a tricky business, nothing is more harmful to a product manager than dirty data, in other words, bad data.
From manual errors to data entry or incorrect observations from focus groups, inaccurate data could have severe consequences on the future of your product. It is up to the product manager to ensure that they sift through the good from the bad and validate that the information gathered is accurate, convincing, and tells a story.
{{ai-powered-solutions-banner-4="/attributes/blog-components"}}
What does the future hold?
Building efficient internal data collection and consumption capabilities is proving to provide a massive competitive advantage to leading enterprise businesses. A decade ago, Barack Obama famously used data analytics to track voter patterns and understand user behaviors to optimize his campaign strategies and drive user donations. Coca-Cola’s marketing teams rely on data analytics daily to serve the right content to their audience at the right time.
The list goes on and on; history is awash with corporations that use data to guide their decisions. As product managers, it is imperative that you embrace data and add that as an ingredient to your product management armory.
Data Analytics is here to stay and the sooner you absorb that into your product, the better. Everyone out there is already utilizing this, so why shouldn’t you?
Ready to fuel your digital product development with AI-powered innovation?
Join our complimentary 3-hour AI Ideation workshop today!
Collaborate with our experienced Data & AI experts to brainstorm high-impact AI use cases that enhance your digital products and drive your business objectives forward.
Don't miss this opportunity to explore the art of the possible and ignite your enterprise business with intelligent applications.